With a dizzying array of new data sources and incessant hype surrounding ChatGPT and its lookalikes, the investment-management industry is buzzing about the transformation potential of generative artificial intelligence (AI). While there’s plenty of low-hanging fruit to exploit, new capabilities also bring new challenges, and we think applying a consistent framework can help.
An Intuitive Framework for Decision-Making
Generative AI, a subset of the AI toolset in data science, uses groups of algorithms to create new content. Generative AI tools are extremely powerful, but they may not be the most effective—or even an appropriate—solution for every problem. In data science, no tool should be a hammer in search of nails.
As we like to say, data science efforts should always start with a question, and defining these early in the process is a great way to focus research. Also, an intuitive, widely applicable decision-making framework (Display) can help investors address issues and determine the right tools for each use. While every day seems to bring new tools and techniques, the approach to making decisions is the same.
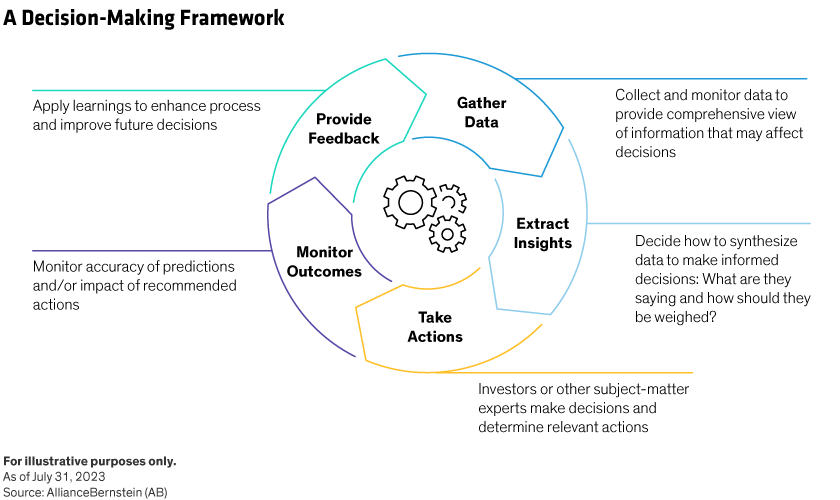
The framework itself is straightforward. Once a question or issue is identified, decision-makers gather a swath of data to gain a comprehensive view. After synthesizing that information and extracting insights, they reach a decision and take the appropriate actions that follow from that decision. Monitoring outcomes ensures that learnings are applied to refine the process continually.
Whether decisions are investment-related or pertain to business operations, most, if not all, of them leverage this framework. That makes it a useful way to assess the effectiveness of developments in a rapidly evolving field, including a proliferation of data sources and tools.
Evaluating a New Wave of Data Sets and Tools
Over the past decade, the investment-management industry has seen a huge influx of new data sources and more sophisticated analytical tools. The tools include generative AI, which is designed to create new content or data—not just analyze or process existing data.
These newcomers have enabled data scientists to sharpen their game. Timely data such as foot traffic and online inventories, for instance, can help investors proxy company revenues, enabling them to assess fundamentals faster than they used to. And powerful generative AI capabilities can be leveraged to generate code and text across a variety of business processes.
While machine learning is very effective at extracting insights during the decision-making process, generative AI is a much more robust tool. It plays a sizable role in three stages of decision-making: creating synthetic data, extracting insights from data and recommending actions.
Using the framework as a lens, we can assess the capabilities and impact of many tools and techniques, helping investors determine which ones are most relevant.
Getting More Systematic with Decision-Making
Increasingly, investment teams are striving to create repeatable processes to identify opportunities, and both discretionary and systematic managers are using screening tools to streamline processes. For many investors, though, the tools do just that—screen. Human analysts must kick the tires before deploying investment ideas in portfolios, so the “take action” stage of decision-making is still largely people-driven.
Systematic investors are changing this paradigm, creating algorithms that seek to make automated decisions based on inputs. Generative AI pushes the envelope even further, recommending actions or steps across diverse processes. In customer service, for instance, chatbots can handle routine, repetitive and time-consuming tasks. The result? More free time for humans to tackle the complex issues.
Systematizing decision-making is not only efficient, it’s necessary. More data sources, better algorithms and timely responses are demanded in investment decision-making, client servicing and operational processes. To harness this trend, the industry can leverage generative AI, combined with natural language processing (NLP), to make better decisions.
The Alphabet of Low-Hanging Fruit: NLP, NLU, NLG
NLP, as well as natural language understanding (NLU) and generation (NLG), enable models to read text inputs, make sense of them and create responses. Chatbot applications are the most obvious uses, but the applications for investment management are wide-ranging.
NLP excels at handling essential tasks that unlock the potential of text-based information (Display), enabling asset managers to gauge market sentiment, discern trends, identify sales and investment opportunities, extract key data and insights from long documents, and create solutions and responses to issues or questions.
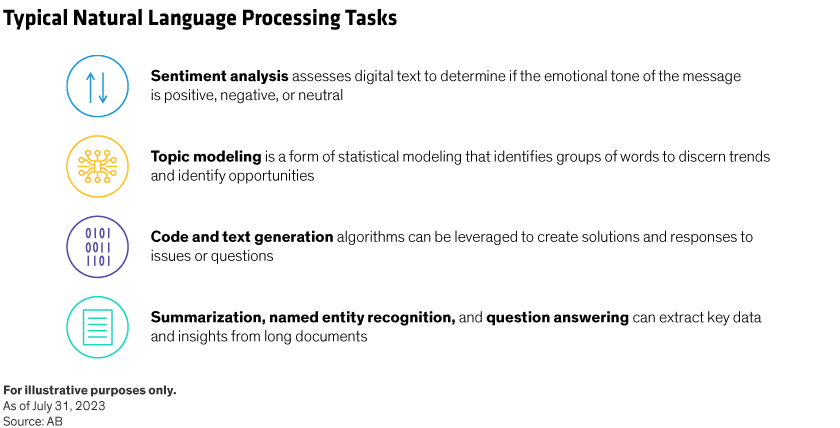
These tasks improve the decision-making process—most directly in extracting insights and taking action. Sentiment analysis is the most obvious example, but NLP tools can also uncover patterns, such as finding textual similarities in articles or generating ideas from raw data. NLP’s most important impact may be its ability to systematically synthesize data to unlock opportunities—efficiently and at scale.
Fine-Tuning and Prompt Engineering to the Rescue
NLP and generative AI no doubt present enticing opportunities, but there’s no shortage of challenges, and plenty of work for asset managers to do. Applying the right human talent is an obvious need, but the tools themselves have wrinkles. Data quality, data privacy and the ability to interpret models are common areas where issues arise, but hallucinations—the tendency of AI to “make up” answers—may be the most troublesome.
That’s where fine-tuning and prompt engineering come in.
Training and tailoring NLP models for the language and nuances of their specific domains can raise the game of these tools. For example, we’ve fine-tuned generic NLP models to assess the prevailing sentiment in financial text. The phrase “creating disruption” may not naturally be viewed as a positive, but to our technology analysts, it could represent an opportunity.
Prompt engineering, an emerging field already making an impact, optimizes inputs into large language models to drive better responses. In the financial world, we find that prefacing our queries with the right context drives better-quality outputs. A simple example would be including “you are a financial analyst” at the beginning of a prompt to an AI model.
Could the Iron Man Model Be the Winning Combination?
As we evaluate new data and tools through the framework, it’s also important to look to the horizon. What kind of investor, salesperson or decision-maker will be successful tomorrow?
Perhaps Iron Man is the inspiration—the integration of a tech-savvy human expert in a specific field, such as financial analysis, with an AI-powered suit of armor that delivers timely, effective prompts to enable decisions. The suit uses all available data to suggest a course of action, but the human can override it based on the circumstances. For example, tomorrow’s “Iron Person” may realize that a market regime change or unprecedented disruptive event like COVID-19 makes today unlike the past, requiring a different response.
Generative AI and NLP can create new content, but their current vulnerabilities suggest that people will still play a critical role in the new world order. Large language models may generate text that sounds reasonable, but human experts must review it before executing on it. The same is true in the investment arena: tools may suggest reasonable investment actions for portfolios, but investors must vet the decision quality and own the outcomes.
Returning to the decision-making framework, generative AI and NLP can gather data, extract insights and even take action effectively and efficiently. But human insight is vital in ensuring appropriate model recommendations and in upgrading and maintaining the quality of signals and actions.
As we see it, the “Iron Person” is tomorrow’s winning combination for generative AI.
The views expressed herein do not constitute research, investment advice or trade recommendations and do not necessarily represent the views of all AB portfolio-management teams. Views are subject to change over time.